From virtual assistants to recommendation systems, the impact of artificial intelligence (AI) is clear. One of the biggest challenges of AI is continuous learning. This means acquiring new knowledge and adapting to new situations over time. However, this advancement is not without its problems. Ensuring that these updates are fair is a challenge. This affects both platform video games and economic and social systems. This article discusses the challenges developers face when balancing learning and fairness in AI.
The challenge of continuous learning in AI
Continuous learning allows an AI to process new inputs without forgetting what it has learned. This problem is known as the “forgetting catastrophe.” Traditional AI systems are trained with static data. This limits their ability to adapt to dynamic changes. In continuous learning, algorithms must integrate new information without affecting previous tasks.
This approach is crucial in changing environments. For example, in video games, where a fair balance between players is sought. Also in financial systems, which must adjust to new patterns without unfairly benefiting certain groups.
Impact on game fairness
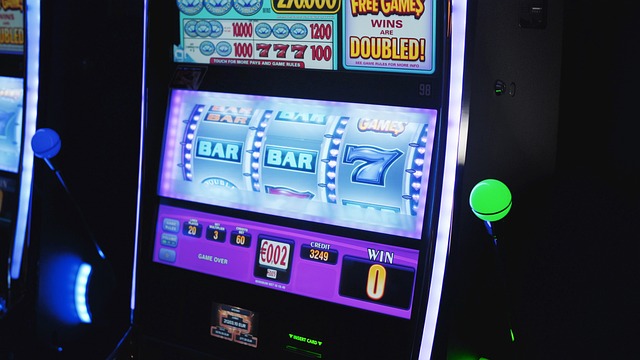
One of the biggest challenges of continuous learning is maintaining fairness. In video games, this means that updates do not benefit certain players over others. For example, if AI learns tactics from advanced players, beginners could be at a disadvantage.
In other contexts, fairness also applies to automated decisions. This includes hiring or lending systems. If recent data reflects inequalities, continuous learning can introduce biases. Therefore, developers must carefully choose update data. In addition, they must process it in a way that does not reinforce or exacerbate these inequalities.
Technical and ethical challenges
Developers face several challenges when implementing continuous learning without losing fairness:
- Selecting balanced data: It is key to use data that fairly represents all groups. This prevents overfitting to dominant groups and encourages diversity in data sets.
- Constant monitoring: Regular audits are needed to identify and correct biases. This includes technical monitoring and the involvement of ethicists and end users.
- Transparency and explainability: AI systems must be understandable. Decisions made by AI must be traceable and explainable, especially in dynamic environments.
- Balancing innovation and stability: When incorporating new skills, it is crucial not to destabilize the system or break its operating rules.
- Resource optimization: Continuous learning demands high computational and storage costs. Reducing these costs without affecting quality is a constant challenge.
Towards fairer continuous learning
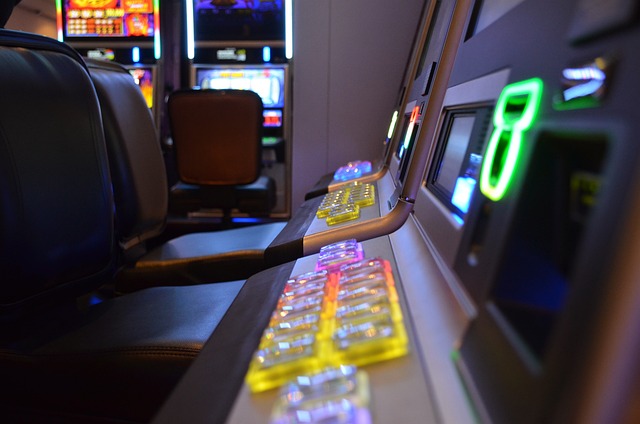
To address these challenges, developers are exploring various innovative strategies:
- Selective memory: Create models that remember key information from the past while learning new things. This avoids the “catastrophe of forgetting” and maintains stable performance.
- Federated learning: Allow models to learn from multiple sources without transferring data directly. This reduces bias and protects privacy.
- External regulation: Work with regulators, experts, and communities to define ethical standards. This ensures responsible development and use of AI.
Continuous Progress
Continuous learning can make AI a more adaptable and versatile tool. But this advancement brings great challenges. Improving its learning capacity without compromising fairness requires care. It is necessary to combine technical innovation with ethical commitment. Only with interdisciplinary collaboration and responsible oversight can a balance be achieved. In this way, technological progress will be compatible with justice in an AI-driven world.